DeepSeek R1 : What is it?
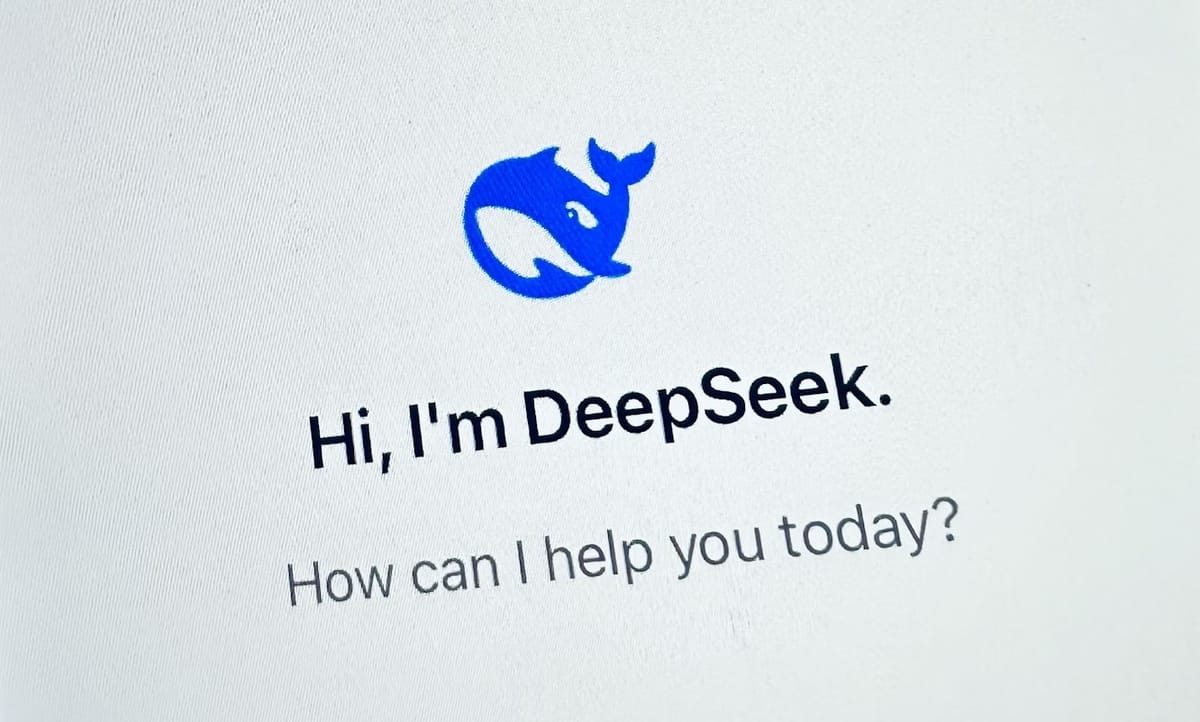
DeepSeek R1, developed by the Chinese AI startup DeepSeek, represents a significant advancement in artificial intelligence, particularly in reasoning capabilities. This model has garnered attention for its performance, efficiency, and open-source nature, positioning it as a notable competitor in the AI landscape.
Technical Development: DeepSeek R1
DeepSeek R1 is an open-source reasoning model that matches the capabilities of leading AI models, such as OpenAI’s o1, but is developed at a fraction of the cost. Trained on approximately 2,000 Nvidia H800 GPUs, the model’s development cost was around $5.6 million, significantly lower than the investments made by Western counterparts. This efficiency in training has raised questions about the vast expenditures in AI infrastructure by U.S. companies, which are projected to reach $310 billion in 2025.
Domain Impact
DeepSeek R1’s development impacts several areas of technology:
• Artificial Intelligence and Machine Learning: The model’s advanced reasoning capabilities push the boundaries of what AI can achieve, particularly in logical inference and problem-solving.
• Natural Language Processing (NLP): Its proficiency in understanding and generating human-like text enhances applications in chatbots, virtual assistants, and automated content creation.
• Computational Efficiency: The model’s development showcases a more resource-efficient approach to training large AI models, potentially influencing future AI research and development methodologies.
Technical Scope
This analysis delves into the technical architecture of DeepSeek R1, its training methodologies, performance metrics, and the challenges encountered during its development. It also examines the model’s integration potential within existing systems and its scalability.
Target Understanding
Readers will gain a comprehensive understanding of DeepSeek R1’s technical foundation, including its architecture and training process. The article will elucidate the model’s practical applications, performance benchmarks, and the implications of its open-source nature on the AI community.
Industry Context
DeepSeek R1’s emergence has significant implications for the AI industry. Its open-source release under the MIT license allows for widespread use and adaptation, potentially accelerating innovation and collaboration within the AI community. The model’s efficiency challenges the prevailing notion that cutting-edge AI development necessitates substantial financial and computational resources, prompting a reevaluation of investment strategies in AI infrastructure.
Introduction to Innovation
DeepSeek R1 marks a pivotal development in AI, introducing a model that rivals leading counterparts in reasoning tasks while being more resource-efficient. Technically, it demonstrates that high-level AI performance can be achieved without extensive computational resources, a notion that could democratize AI development. The industry has reacted with a mix of admiration and concern, as evidenced by significant impacts on global tech stocks following its release.
Technical Background
DeepSeek R1 is built upon advanced machine learning techniques, particularly in reinforcement learning (RL). Historically, developing models with strong reasoning capabilities required supervised fine-tuning with labeled data. DeepSeek R1-Zero, a precursor to R1, achieved reasoning proficiency using pure RL without labeled data, though it faced challenges in readability and language consistency. To address these issues, DeepSeek R1 employed a multi-stage training process, combining supervised fine-tuning with RL to enhance both reasoning and language generation capabilities.
Technical Analysis
The architecture of DeepSeek R1 is based on a transformer model with 671 billion parameters. Its training pipeline incorporates two RL stages aimed at discovering improved reasoning patterns and enhancing performance. The model was trained using 2,048 Nvidia H800 GPUs, highlighting its computational efficiency. Security considerations include ensuring the model’s outputs are safe and align with ethical guidelines, particularly given its open-source nature. Integration challenges involve adapting the model for various applications, considering its scale and computational requirements.
Practical Impact Assessment
For developers, DeepSeek R1 offers a powerful tool for applications requiring advanced reasoning, such as complex problem-solving and decision-making systems. Its open-source availability under the MIT license facilitates integration into various projects without licensing constraints. Operationally, the model’s efficiency reduces deployment costs, making it accessible to organizations with limited resources. However, the scale of the model necessitates careful resource planning for deployment and maintenance.
Industry Implications
The release of DeepSeek R1 has disrupted the AI industry, challenging established players by demonstrating that high-performance models can be developed with fewer resources. This development may lead to increased competition and innovation, as organizations seek to optimize their AI development processes. The open-source nature of the model encourages collaboration and could accelerate advancements in AI research. Businesses may need to reassess their AI strategies, considering the potential for more cost-effective solutions.
Implementation Considerations
Organizations looking to adopt DeepSeek R1 should consider migration strategies that account for the model’s scale and computational requirements. Integration approaches should ensure compatibility with existing systems and workflows. A thorough risk assessment is essential to address potential security and ethical concerns. Resource planning should include provisions for computational infrastructure and expertise in managing large-scale AI models. Timelines should account for the complexity of integration and testing phases.
Future Prospects
Looking ahead, DeepSeek R1 sets a precedent for developing efficient, high-performance AI models. Potential challenges include maintaining model alignment with ethical standards and managing the open-source community’s contributions. Opportunities exist in refining the model for specific applications and exploring further optimizations in training methodologies. The industry may move towards more collaborative and resource-efficient approaches, with an emphasis on open-source development.
Here are the sources referenced in the analysis:
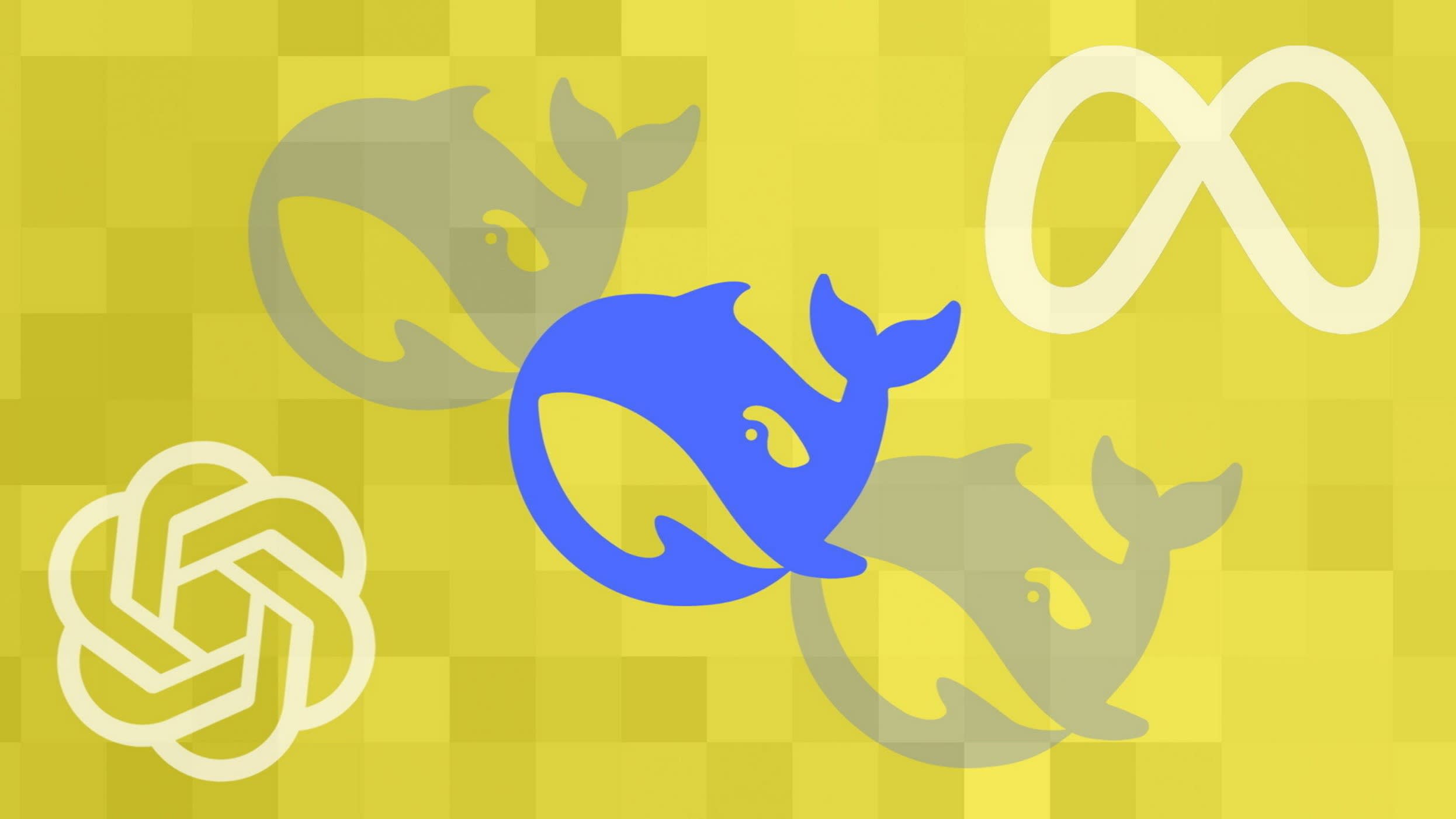
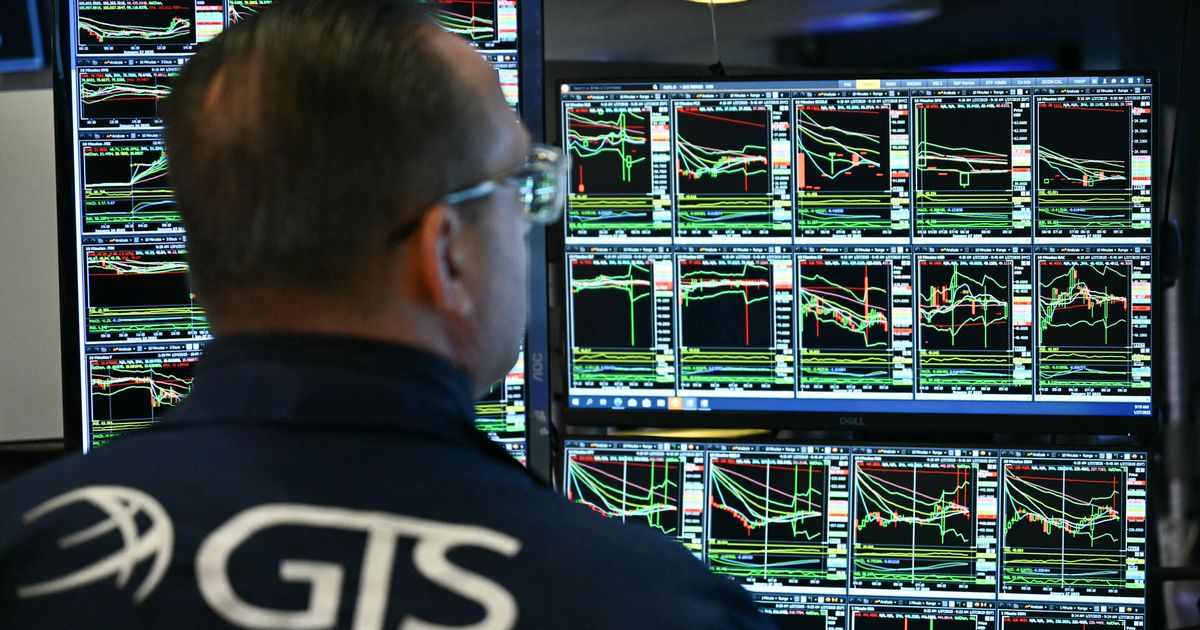
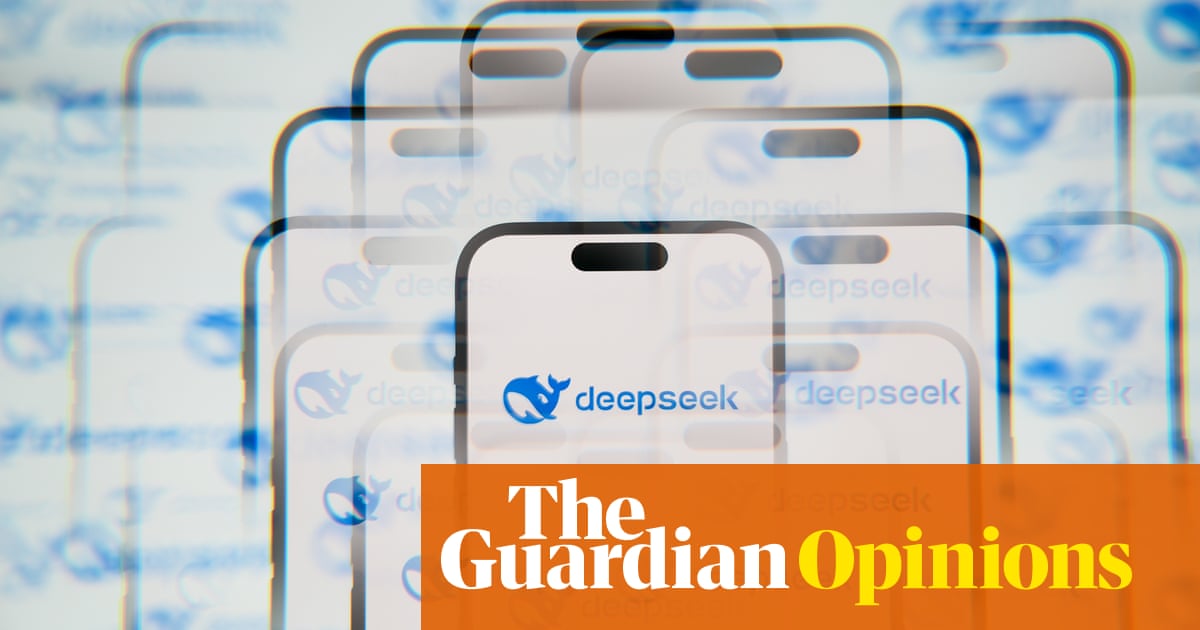
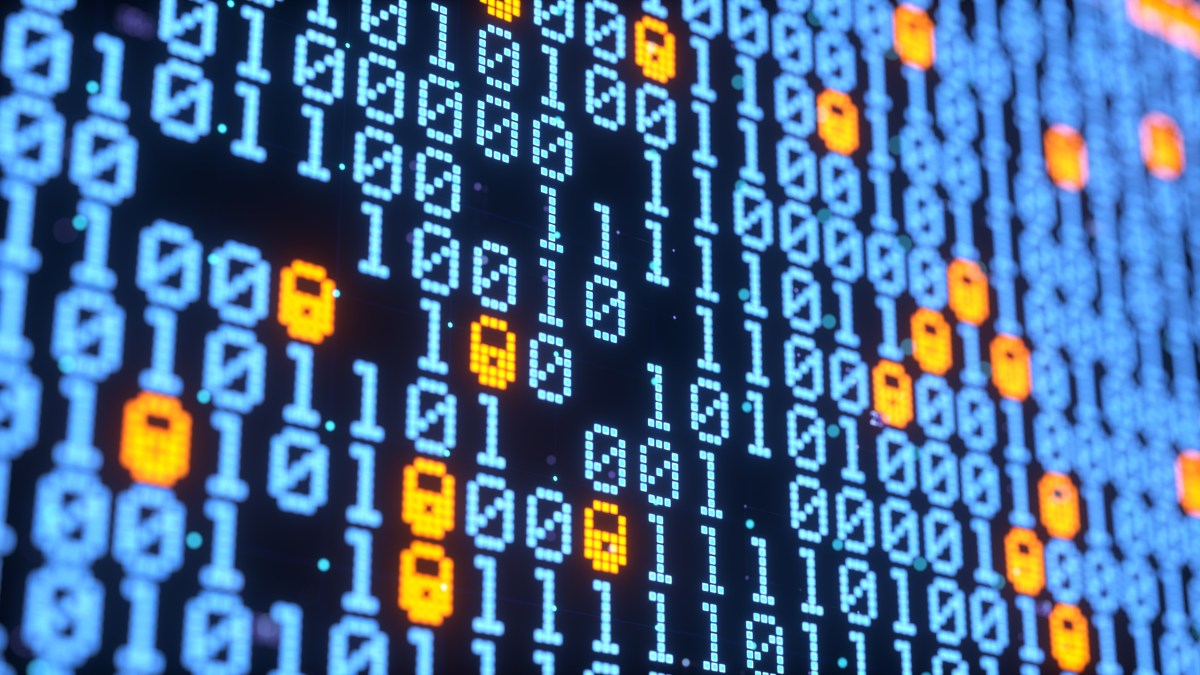
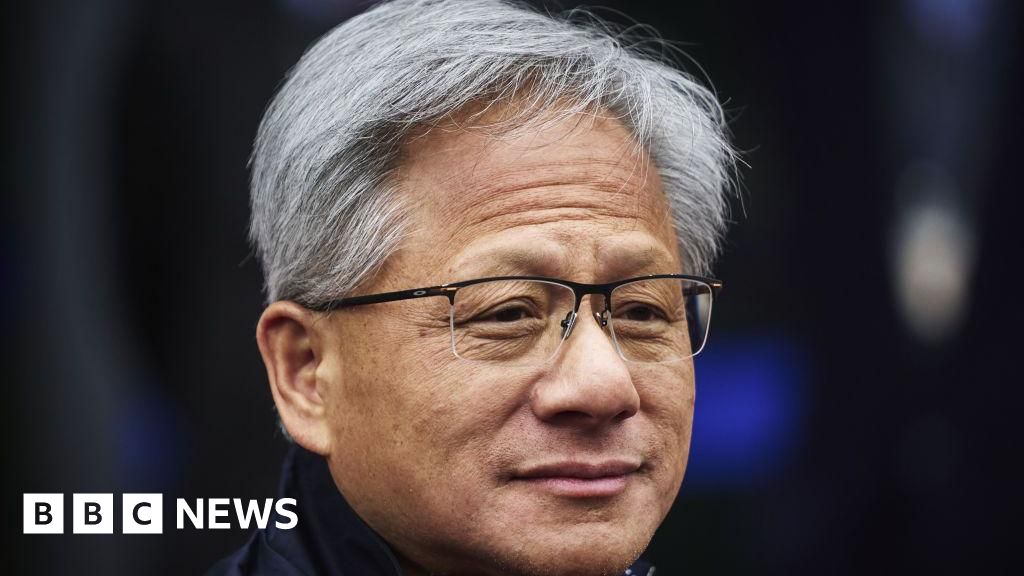
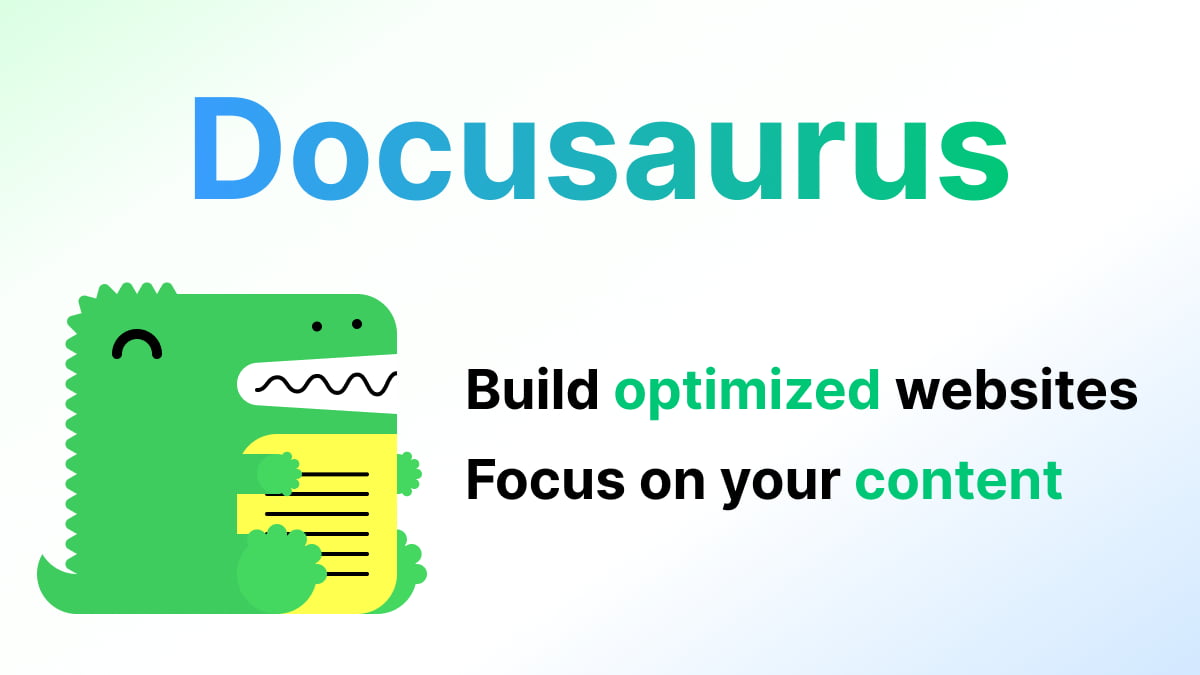
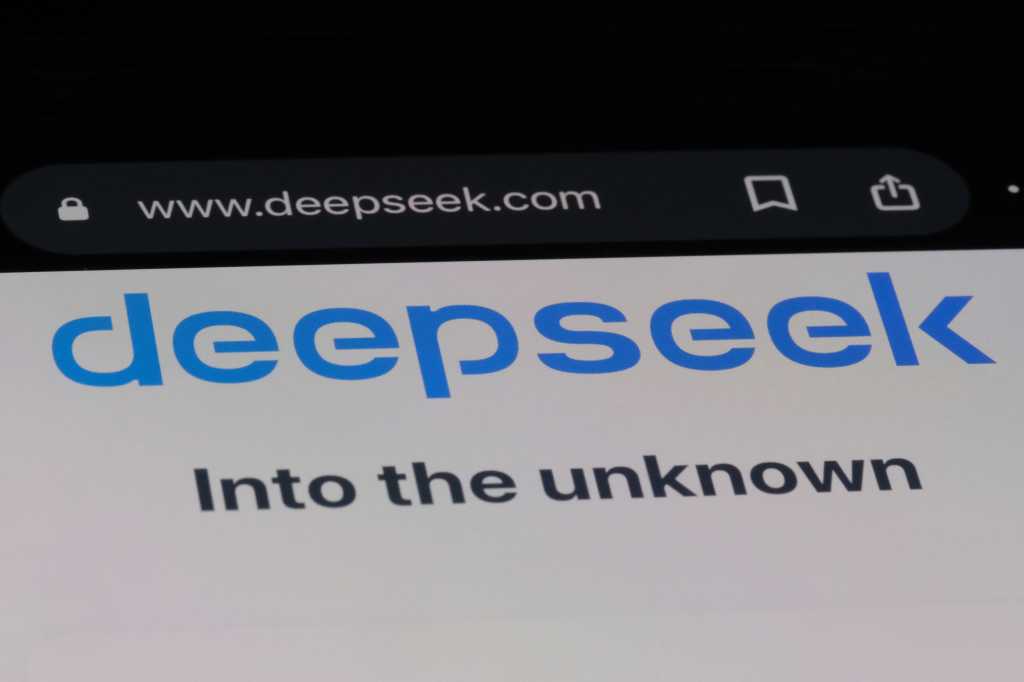
